- Ruhr-Universität Bochum
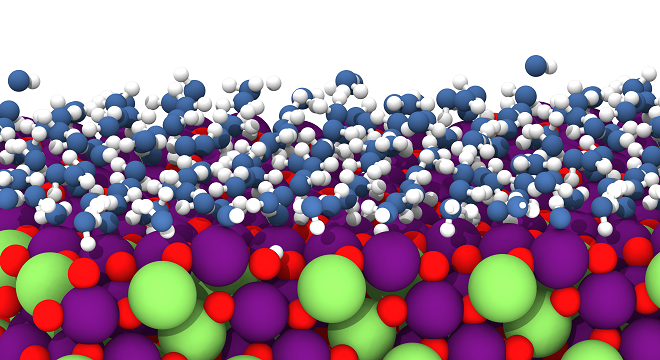
Atomistic simulations with high-dimensional neural network potentials
Today, computer simulations are the key to advances in many fields, from chemistry via physics to materials science, because they can provide important atomic-level information complementing experimental data. The reliability and predictive power of these simulations crucially depend on the quality of the description of the atomic interactions, which determine the properties and reactivity of molecules and materials. While accurate electronic structure methods can in principle provide these interactions directly based on the laws of quantum mechanics, the computational costs of such calculations are prohibitively high and applications are restricted to small systems even when the most powerful supercomputers are used. Simple empirical force fields, on the other hand, have been successfully used for decades to study large systems containing thousands of atoms, but they lack the required accuracy for highly reliable simulations and in some cases can yield even qualitatively incorrect predictions.
Since about two decades we are working on the development of methods to overcome this dilemma by combining modern machine learning algorithms with quantum mechanical electronic structure calculations with the aim to "learn" the atomic interactions, i.e., the shape of the potential energy surface. The resulting machine learning potentials are able to provide the multidimensional potential energy surface, which contains all information about the atomic interactions, at strongly reduced computational costs while the reliability of quantum mechanics is maintained. In this way they allow to perform predictive molecular dynamics simulations beyond the system size that is accessible by conventional ab initio molecular dynamics. An important step in the development of machine learning potentials for complex condensed systems has been our development of high-dimensional neural network potentials in 2007 [1, 2], which can now be termed second-generation machine learning potentials [3]. The key idea of these potentials is to represent the total potential energy of a system as a sum of environment-dependent atomic energies. The local geometric environments need to be transformed to a set of translationally, rotationally and permutationally invariant features that are suitable as input for neural networks.
To achieve this goal, we have developed so-called atom-centered symmetry functions [1], which meet all these requirements. However, for some systems also long-range electrostatic interactions beyond the local atomic environments are important, which can be explicitly included in third-generation high-dimensional neural network potentials [3]. Here, the atomic partial charges are learned by atomic neural networks as a function of the chemical environment. In a second step they can then be used to compute the long-range interactions without truncation based on Coulombs law. In the fourth generation of high-dimensional neural network potential, a global charge equilibration step is employed to determine the global charge distribution in the system. This enables the inclusion of non-local phenomena like long-range charge transfer, which is omnipresent in many molecules and materials. Thus, over the years, the capabilities of machine learning potentials, which are now a very active field in the area of atomistic simulations with many different and very accurate methods being constantly proposed, have substantially grown and step by step the complexity of systems, which can be studied with first principles accuracy, has been extended.
Apart from our focus on method development, in our group we have applied high-dimensional neural networks to many different types of systems including materials like silicon, carbon and copper, but also oxides like zinc oxide and metal-organic frameworks. As part of the cluster of excellence RESOLV, we are particularly interested in aqueous systems, like liquid water and electrolyte solutions, but in particular also solid-liquid interfaces, which are of central importance for catalysis and electrochemical energy storage and conversion. An example is the solid-liquid interface of the lithium intercalation compound LiMn2O4 depicted in Figure 1, which is of interest for catalytic water splitting. Recently, in November 2022, our group has moved to Ruhr-Universität Bochum, to establish the new Chair for Theoretical Chemistry II as part of the Research Center Chemical Sciences and Sustainability of the Research Alliance Ruhr. We are now looking forward to many fruitful collaborations in the very dynamic and active research environment in the Ruhr area, which offers a plenty of opportunities to further improve and apply our methods.
References:
[1] Behler J. and Parrinello M., Physical Review Letters (2007), 98, 146401.
[2] Behler J., Angewandte Chemie International Edition (2017), 56, 12828.
[3] Behler J., Chemical Reviews (2021), 121, 10037.
[4] Eckhoff M. and Behler J., Journal of Chemical Physics (2021), 155, 244703.