- Ruhr-Universität Bochum
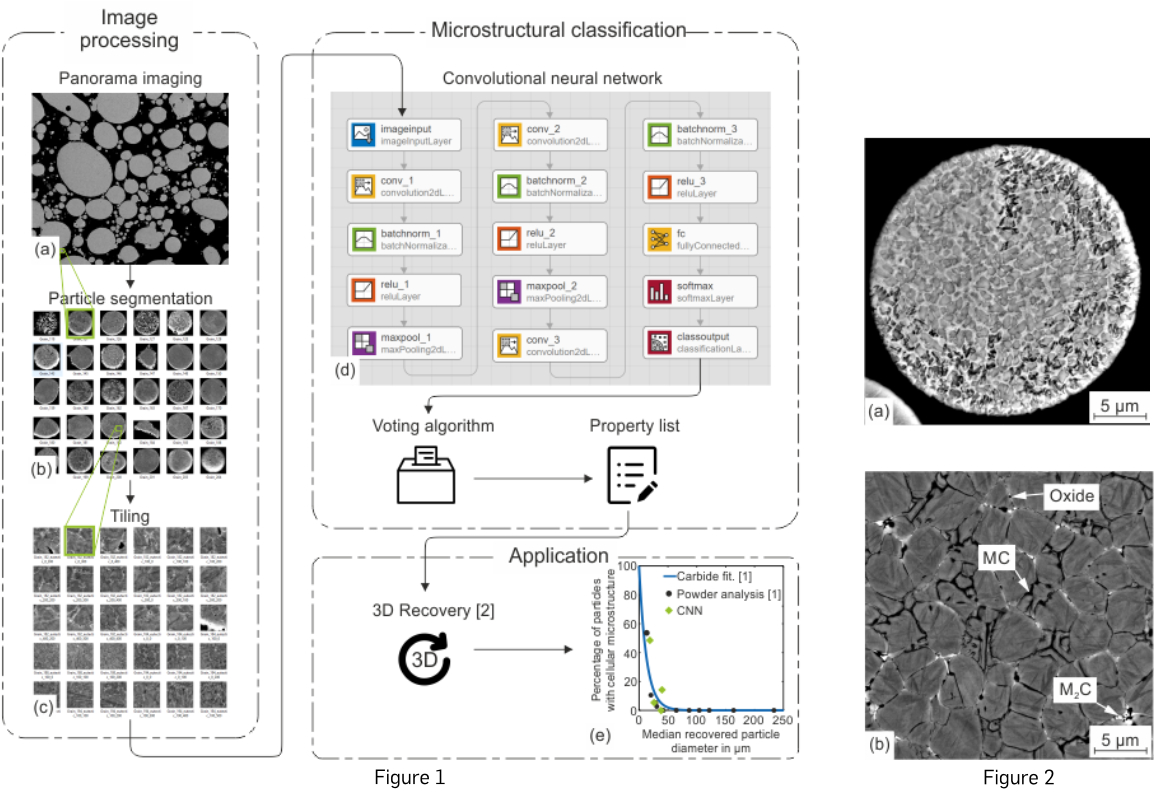
Using Convolutional Neural Networks for Fast and Reliable Microstructural Analysis
At the Chair of Materials Technology (LWT), we dissect the relationships that permeate and define material selection and design. Correspondingly, the observation, description, and ultimate prediction of causal connections between processing and emerging macroscopic properties stand at the heart of our activities. This exercise is deductive and traditionally bases all associations on physical parameters and their interpretation. For instance, we routinely investigate the effects of manufacturing variables on the resulting mechanical properties, such as hardness, toughness, or fatigue life. To that end, the microstructure is the subject of intense examination, as it is ultimately responsible for the observed emergent behavior. Many of the scientific or technical questions that we strive to answer boil down to quantitatively studying the—sometimes subtle—effects of processing on the microstructure in terms of known or hypothesized thermodynamic and kinetic phenomena. Then, we scrutinize the microstructure as the thermomechanical interaction of its microconstituents to explain observed or expected measurable macroscopic properties.
Incorporating Data Science and Informatics into the rigorous framework of Materials Science and Engineering is the quintessence of Integrated Computational Materials Engineering and Materials Informatics. In Materials Engineering, the challenge lies in developing information-based principles that emphasize physical meaning. In other words, technological applications set feasibility boundary conditions that cannot be circumvented. Data-based methods require careful adaptation and implementation to ensure applicability and relevance in application-oriented research.
In this short article, we present one of our efforts toward increasing speed and reliability in repetitive microstructural analysis tasks through machine learning. Microstructural characterization represents a prime candidate activity because of three reasons: Firstly, micrographs are nothing other than data arranged in matrices. Secondly, large imaging campaigns produce heaps of images. And, thirdly, we can embed these automatic analyses directly in existing workflows without ever straying from standard physical descriptors.
Figure 1 presents the proposed approach. In a nutshell, we trained a convolutional neural network (CNN) to recognize the textures of the solidification structures in powder samples of the high-speed steel PM HS 3-3-4, depicted in Figure 2. Due to the extreme cooling speeds of the smallest steel droplets, they solidify into the very fine cellular microstructure exemplarily displayed in Figure 2(a). In contrast, Figure 2(b) shows a more massive droplet with regular eutectic solidification and the corresponding eutectic carbides. Telling these mechanisms apart is technologically relevant because they produce distinct carbide morphologies when the powder is processed in the hot isostatic press to a semi-finished product. To complement the CNN, we added the particle segmentation and tiling steps and included a voting algorithm that democratically decides the solidification mechanism based on the decision on the individual tiles. These processing stages are detailed in Figure 1(a)-(c). With the trained CNN architecture in Figure 1(d), we are able to automatically ascribe each of the 1500 powder particles in one square millimeter area to either category in a matter of minutes. In Figure 1(e), we present a use case. We expanded each segmented particle's property list to include its equivalent area diameter besides the solidification structure. With the help of a three-dimensional size recovery algorithm, we could reproduce the trends in a fraction of the original time.
At LWT, we actively explore original ways to generate new knowledge and efficiently improve existing exercises. As stated above, this brief account serves as an example—if you are interested to know more, visit our webpage: www.wtech.rub.de or get in touch with the authors!