- Ruhr-Universität Bochum
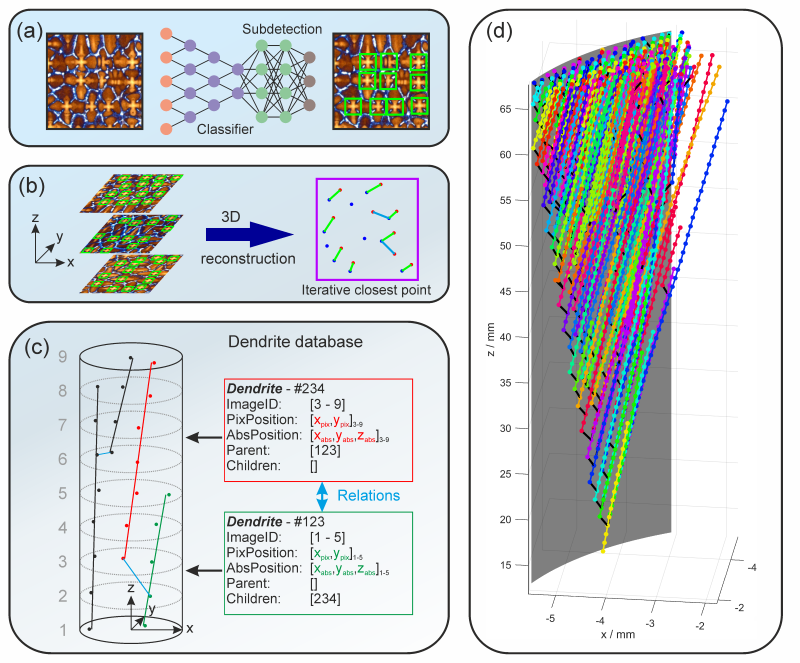
Microstructure Informatics
Quantitative characterization methods of material microstructures represent important tools in materials science. A solid understanding of microstructural features is required to develop suitable models which help to understand the relations between manufacturing, morphology and performance of engineering materials. In recent years, the capability and reliability of advanced computer vision techniques has drastically improved. Artificial neural networks, as well as machine and deep learning approaches have proven their potential in different fields of science, e.g. in applications for autonomous driving cars. Similar methods can be adapted for quantitative image analysis in material scientific projects where they can be used to automatically characterize different types of microstructures with an accuracy that outperforms classical algorithmic computer vision approaches.
One interesting use case for automatic image analysis based on deep learning techniques is the 3D characterization of dendritic microstructures in single crystal nickel based super alloys. Directional solidification is a key processing technique for today’s superalloys. The evolution of the cast microstructure is accompanied by the competitive growth of dendrites, leading to the formation of crystal mosaicity. Crystal mosaicity is characterized by small misorientations between neighboring dendrites. During solidification, interactions between growing dendrites (e.g. extinctions of primary or branching of tertiary dendrite arms) determine the morphology of the cast microstructure and can thereby influence the high temperature creep and fatigue resistance of the material.
To gain a better understanding of the geometrical details of the interactions and how they affect the as-cast microstructure, a three-dimensional characterization process is required, where individual dendrites are traced over a certain distance of solidification . For this purpose, we developed an automatic characterization routine with four characteristic features: (1) A tomographic serial sectioning procedure, where equidistant cross-sections are first cut out of the cylindrical single crystalline specimen and then characterized by optical microscopy. (2) A deep learning object detection architecture consisting of a classifier and a subdetection network, which locates the coordinates of the primary dendrite arms, Fig. 1a. (3) An image registration routine based on an iterative closest points algorithm to reconstruct the 3D growth paths of dendrites in space, Fig. 1b. And (4), a 3D dendrite specific data base capturing all geometric properties and interactions between the dendrites, Fig. 1c. In this way we effectively created a digital twin of our dendritic sample which can now be used to extract morphological information in an automated fashion. This allows us to investigate interactions between multiple dendrites as well as interactions between dendrites and the mold wall during solidification, Fig. 1d. Furthermore, overall statistical properties of the complete dendrite population in terms of the evolution of dendrite spacings, the frequency of extinctions and branching events and the effect of misaligned dendrites on their surroundings, can be extracted. This approach allows for an efficient automatic exploration of microstructural properties and sets us up for future utilization in terms of big data mining.