- Ruhr-Universität Bochum
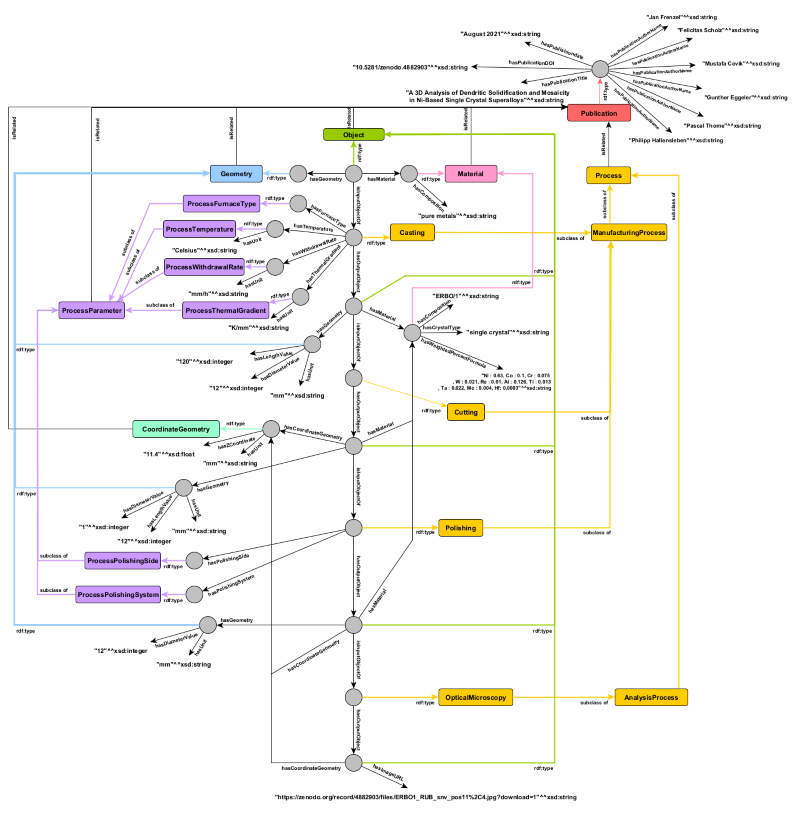
A Knowledge Graph for Superalloys
The main mode of communicating new scientific knowledge was and still is the academic peer-reviewed paper. Data is presented in various forms: micrographs, functional dependencies (x/y plots), photographs, etc. Knowledge is communicated by providing an introductory context in written form with references to other papers, a presentation of the used equipment, materials, and methods (reproducibility), and the resulting measurements including their description (results), again in text form, typically followed by a discussion that extracts principles, relationships and provides generalizations. Finally, the most important findings and their consequences are concluded and possible limitations are stated. This is one of the best ways (we know of) to communicate knowledge to humans. The knowledge is, however, not machine-readable in general. “Machine-readable” refers to the possibility of (semi-)automated access to the data and contained knowledge through algorithms querying a data source.
The Collaborative Research Centre (CRC) Transregio 103 “From atoms to turbine blades – a scientific basis for a new generation of single crystal superalloys” is currently in its last funding phase. Results from more than a decade of research are already published in more than 300 papers with more to come. This body of literature constitutes the scientific output of the CRC, accessible to humans. Links between the papers exist through citations, but the actual knowledge from these papers is not linked and not machine-readable and, therefore, less accessible to further scrutineering. To create a knowledge graph of the accumulated knowledge, we present one step in the digitalization process of the knowledge using already published experimental work.
A knowledge graph consists of the data itself and a data model (ontology) which defines how individual data are connected. This knowledge graph can then be queried with computers for analytics (How many measurements exist for a given alloy? Are there connections between measurements that have not been exploited?) but also serves as an entry point for further analysis to infer hidden latent knowledge through various Materials Informatics methods. As a first step towards the creation of a full knowledge graph of all the papers published in the CRC, we devised a protocol to formalize the knowledge contained in Scholz et al. through a paper-specific ontology. Figure 1 shows the developed ontology. It includes bibliographic information, chemical composition, all described processes for sample preparation, and finally the analysis process which led to the published results and conclusions. The result of this protocol is a digital representation of the data and, most importantly, how this data was obtained (metadata) by Scholz et al.. But for a knowledge graph to be useful for Materials Informatics approaches, it has to contain the knowledge of many more papers.
Our current protocol requires a substantial amount of manual work to read the paper, structure the information, and translate it into an ontology. This manual process is very instructive but does not scale to the over 300 articles published in the context of the CRC. Two solutions are required in the future for knowledge representation. One for already published work, as demonstrated here, and one for the future publication of findings. The former involves the development and deployment of tools from the field of natural language processing and text-parsing tools to automate the extraction of information from text-based data. This allows to automatic processing of knowledge in text form which can then be included in an existing knowledge graph. The latter requires fundamental changes in how data is (experimentally) recorded and annotated through digital event logging, electronic lab notebooks, as well as a research data management system and is a work in progress in many communities in Materials Science and Engineering.
Link to online version of the ontology with an automatic layout